Nihal Nayak
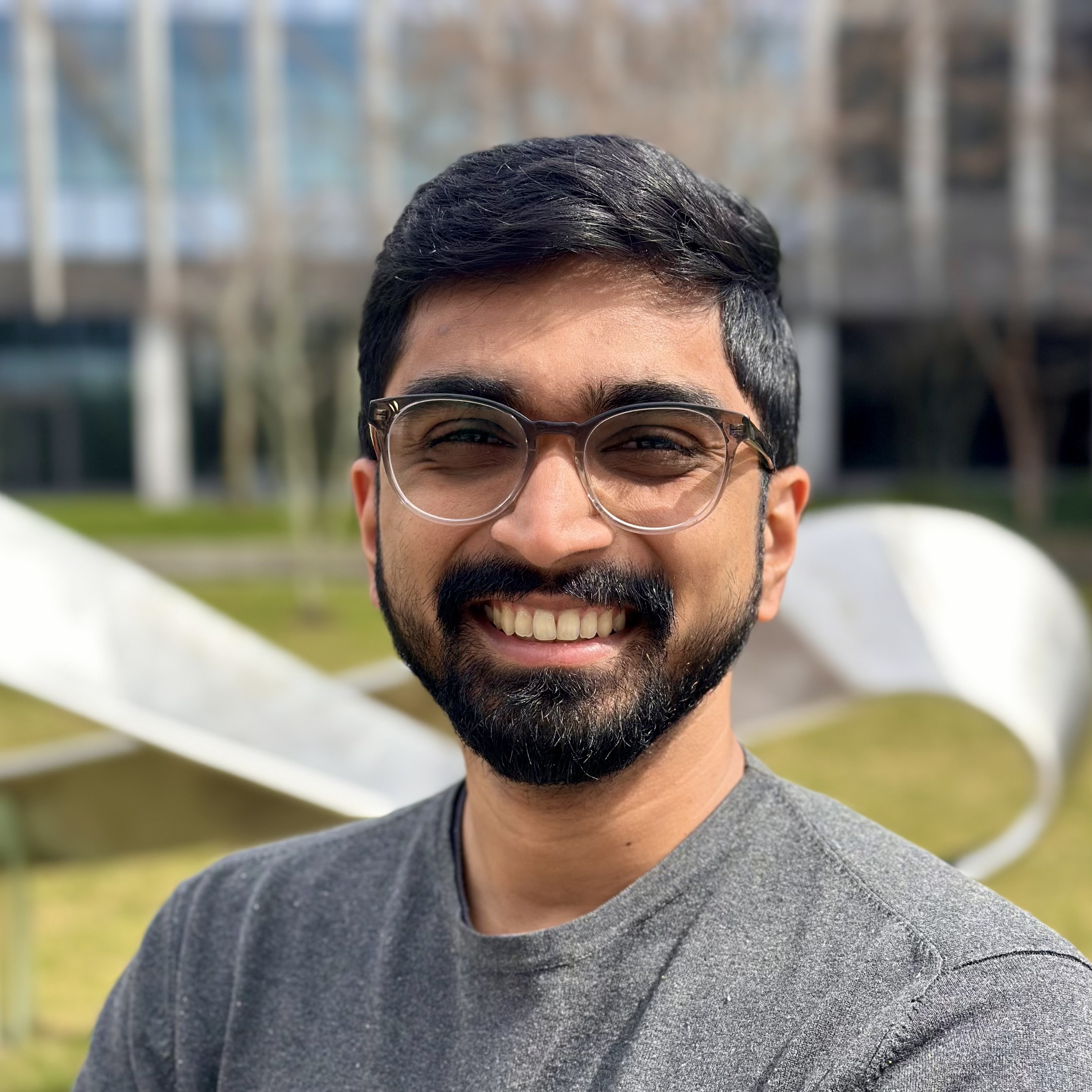
Update: I’m on the job market! Reach out if you think I’d be a good fit in your organization.
One-Page Resume | CV (Updated in Jan 2025)
I am a Ph.D. candidate in Computer Science at Brown University. I work with Stephen Bach. My research focuses on building zero-shot systems, a class of intelligent systems that generalize to new classes, tasks, and environments without human annotations. My approach involves identifying the limitations of various components in zero-shot systems, such as large language models (LLMs), and improving them with data-centric solutions.
Here is a summary of my recent research:
-
Synthetic Data Generation. Introduced Bonito, an open-source model that converts unannotated text from specialized domains into instruction tuning datasets to adapt large language models without annotations (ACL Findings, 24).
-
Composition. Introduced compositional prompt tuning, a new parameter-efficient prompt learning method that learns to decompose classes into sub-concepts and recomposes them at test time for improved zero-shot performance (ICLR, 23). Next, created a synthetic benchmark to systematically study compositionality in vision language models. We find that they often fail to generalize to compositions that require binding (EACL Findings, 24).
-
Structured Knowledge. Created ZSL-KG, a general-purpose zero-shot learning framework with a novel transformer graph convolutional network (TrGCN) to learn class representation from common sense knowledge graphs (TMLR, 22).
Email : nnayak2 [at] cs [dot] brown [dot] edu
news
Oct 30, 2024 | Excited to share a new pre-print on pre-training foundation models in Academia. Featured in Nature. |
---|---|
Jun 5, 2024 | Excited to share that Bonito was accepted to ACL Findings 2024. |
May 3, 2024 | Spotlight talk at NeNLP and invited talks at Snorkel (Video) and Amy Greenwald’s Reseach Group on Bonito. |
Feb 27, 2024 | Excited to share new preprint on adapting large language models to tasks in specialized domains using Bonito, an open-source model that converts raw, unannotated data into instruction tuning datasets. |